10 predictions for Customer Data Platforms (CDPs) in 2030
How up-and-coming trends will transform CDPs by 2030
I’ve learned the hard way over the course of my career in software and data that designing and managing a great customer experience is hard. No two customer experiences are the same and they each have their unique background stories. Whenever we try to simplify and model experiences linearly, we always fall short. Customers rarely follow “one path”; every customer arrives at your product or service with a different context, a different struggle and through a different medium.
Recent developments in Customer Data Platform (CDP) — from the likes of Segment and Rudderstack — are starting to address these issues. But I think there’s still a lot more to come as we move closer to the end goal of offering a great, delightful customer experience. In this post, I’ll paint a picture of what I hope and expect to see from CDPs by 2030. I’ll explain what a CDP is and provide extra context on the data landscape, before diving into my predictions (expectations?) for 2030.
So what’s a CDP?
Let’s first get definitions out of the way. What’s a customer data platform? David Raab, the founder of the CDP Institute, describes it as follows in his 2013 blogpost titled I’ve Discovered a New Class of System: the Customer Data Platform —
…systems that gather customer data from multiple sources, combine information related to the same individuals, perform predictive analytics on the resulting database, and use the results to guide marketing treatments across multiple channels. — David Raab, Founder at CDP Institute
And a more concise definition from CDP Institute:
…packaged software that creates a persistent, unified customer database that is accessible to other systems. — CDP institute
“Packaged software” here pertains to pre-built software that can be set up and maintained without the level of technical expertise needed to manage data warehouses on your own. “Persistent, unified customer database” refers to the primary value proposition of CDPs, which is the ability to associate events from multiple sources to a single user profile. In other words, CDPs tie all the touch points in a customer journey to a single user profile. “Accessible to other systems” ensures that the CDP doesn’t live in a silo and insights can be readily deployed. These properties of a CDP, at least in theory, allow us to follow an individual customer’s journey from the first engagement to the moment of purchase and beyond.
Where we are today…
Over the last years, we’ve seen great strides towards understanding the customer and capturing data to improve customer experience. We’ve slowly but surely removed ourselves from the aggregate nature of the likes of Google Analytics and started to collate data from various sources — in vast volumes — to build meaningful customer profiles. In the early 2010’s, when we had a plethora of analytics software spring up — the Mixpanels and Amplitudes of the world — Segment’s fan-out architecture was a breath of fresh air that consolidated the then-fragmented data collection. Segment created a single interface through which to capture data points from various data sources. We plugged Full Story, Mixpanel and a bunch of other tools into Segment, so that we could start creating a more holistic and multi-angled view of our customers. With large volumes of data flowing through Segment, nifty features emerged, such as data replay that let us playback historical data to other SaaS tools. Granular data stored in Segment also allowed us to build “Personas” and follow customer journeys at an event-by-event level. In 2020, Segment was acquired by Twilio for $3.2 billion [*], an astonishing feat for a YC startup that initially struggled and almost quit in 2012 before breaking through with their successful Hacker News post.
While Segment made steady gains, a new buzzword — the “Modern Data Stack” (MDS) — also gained ground. The MDS is a group of cloud tools that together form a data platform centred around a cloud data warehouse. A typical constellation of the MDS may look like so: Fivetran to collect data from various data sources (data integration); AWS Kinesis to provide the backbone of real-time data (data streaming); AWS S3 to store raw data (data lake); DBT to transform and model data (data transformation); AWS Redshift to act as the central data warehouse. Some platforms such as Snowflake package some of these value propositions into a single service. In the recent past, we’ve seen Reverse Extract-Transform-Load (ETL) tools, such as Hightouch, join the MDS ecosystem. These tools extract rich customer insights from the data warehouse and place them directly in tools used by sales, marketing and operations. The MDS constellation, where the data warehouse sits in the middle, has become increasingly common as hosting your own data warehouse has become dramatically more affordable. The trend has meant that even startups — where I’ve spent most of my career — can now afford to store and gain insights from large quantities of granular data. It’s the comet-hits-earth moment for data: when hosting data warehouses becomes negligible, even the smallest businesses can afford to offer great customer experiences. This is exciting!
It is in this context that the likes of Rudderstack are finding new, data warehouse-centric angles to collecting and managing customer customer data. Like Segment, it collects data from various sources and plumbs data points to popular destination platforms where the data can be operationalised. However, Rudderstack gives you more control and is warehouse-centric: you can bring your own data warehouse such as Snowflake, Google BigQuery or AWS Redshift; identity resolution can be done adjacent to your own warehouse.
New solutions like these are cropping up constantly as we continue to refine our solutions for the age-old problem of detecting and mitigating customer issues as well as identifying new opportunities.
What’s coming in 2030?
With the context set, let’s dive into the predictions for 2030. Some are relatively obvious predictions that we’ve already seen some evidence of in the recent past. But other predictions will be pure “finger in the air” extrapolations that may seem whacky today but may be a reality in the not-too-distant future! There’s no way to be completely certain but I’m willing to bet that some of these bets won’t be too far off. Here’s a summary:
- The warehouse is your CDP
- Serverless will cut costs and make warehouse-centric CDPs ubiquitous
- No-Code events will further increase volume of customer data
- The CDP will serve both marketing and data teams
- Identity resolution will be deterministic but probabilistic for certain use cases
- The Metrics Layer will be the norm and CDPs will benefit from it
- SQL will be on steroids
- DataOps tools will ensure 10x trust in data
- Personalised, just like you designed it
- Customer insights at your fingertips, with edge warehouses
Allow me to dig in and explain below.
1. The warehouse is your CDP
This is probably the most obvious. We’re already seeing warehouse-centric infrastructure in the likes of Rudderstack. Hightouch is arguing for the warehouse becoming the new CDP. If your CDP is siloed and behind a “proprietary” wall, there’ll be limited control over your data and limited ability to query against your own customer data. Some CDPs of today evolved into silos in a period when the data warehouse ecosystem was less than mature. All-in-one solutions made sense.
But in the future, as the MDS ecosystem matures, we’ll see more powerful tooling built on top of the warehouse. With warehouses becoming cheaper every year and custom machine learning models becoming easier to deploy on your own data, it’s almost a no-brainer that people will flock to where you can find the best insights: the warehouse-centric CDP.
2. Serverless will cut costs and make warehouse-centric CDPs ubiquitous
The rise of serverless and its arrival in warehousing — the likes of Snowflake, Google BigQuery and AWS Redshift Serverless — has made warehousing even more accessible as you can pay for your data warehouse only for the amount of time you use it. This will mean more businesses that are able to afford warehousing and, of course, warehouse-centric CDPs.
With serverless technology getting faster, we should (hopefully!) see performance improvements in serverless warehousing as well. The fastest growing apps in 2021 in Segment’s ecosystem are in fact BigQuery and Snowflake [Segment], so this trend may hardly come as a surprise to those following the space closely.
3. No-Code events will further increase volume of customer data
No-Code, No-Code. I’m a big believer in No-Code software automating standard business processes that previously needed engineers to implement. Connecting Stripe, HubSpot and Slack has never been easier and No-Code software such as Zapier and Make (formerly Integromat) will continue to make SaaS software easier to stitch together. Of course, the second order effect of such a trend is that the volume of events will increase massively. We’ve only just started to see back-end servers break through the most popular data sources (see Segment’s 2021 CDP report) and I expect No-Code to cause back-end, granular event volumes to explode. With each new API call and webhook performed by No-Code, we’ll have more data points that we can send to our warehouse to distill insights.
Let’s consider an example: your customer Mary discovers your service via a post you wrote on your blog; she signs up to your newsletter, which triggers a series of drip-feed emails; further down the line, she buys your eCommerce product; she gets a confirmation email; the product is dispatched to her; she receives the product and uses it for the first time; there’s a problem and she complains via the support desk. All of these interactions will be powered by No-Code integrations and emit data points that will be processed by a warehouse-centric CDP. The data points may even go through machine learning (e.g. NLP) algorithms to add further context to the data. The CDP will need to make sure that the Convertkit email, Stripe customer, Postmark email, Shopify customer and Zendesk requester are all associated with the single user profile (Mary’s).
The CDP will hold the key to making sure that the support agent has the full customer journey and context in front of them when they address the customer complaint. With more No-Code events, there’ll be a greater need for identity resolution and CDPs.
4. The CDP will serve both marketing and data teams
This is a fairly straight-forward prediction and requires little explanation. CDPs started as a tool for marketing to consolidate data points from disparate sources and make event tracking plans easier to manage for developers. But to really harness your data and distill insights that can be plugged back into your CRM and help desk, you’ll need to get your data team involved.
Warehouse-centric CDPs will make customer experience a truly multi-disciplinary endeavour. The best businesses won’t be the ones only using off-the-shelf, generic customer models offered by siloed CDPs but will be those that have bespoke customer journey models living in their warehouse-centric CDP. Reverse ETL tools — or one step beyond, Data Activation tools such as Hightouch — that integrate with the CDP will undoubtedly expand to finance and other operational use cases [Mikkel Dengsoe].
5. Identity resolution will be deterministic but probabilistic for certain use cases
For identity resolution to really work and to gain a full profile of your customer, you need to have control of your identity graph. The identity graph needs to be adjacent to your warehouse and we’re already seeing this trend in Rudderstack, etc. But with the large amount of touch points that the customer may have in their journey, there’ll be a limit to stitching together user profiles with a rules-based algorithm. With privacy trends and the explosion of touch points, this problem will only get harder. At a certain point, a probabilistic (machine learning?) model will be needed to resolve customer interactions to a single user profile at scale. While this sounds great in principle, probabilistic identity resolution comes with risks. Mistakenly linking two profiles that shouldn’t be linked can lead to irrelevant customer experiences at best (wrong ad shown) and lost customer loyalty at worst (alarm bells ringing when you see someone else’s data).
So how do we get around this? We’ll apply probabilistic resolution for lower stake but higher volume scenarios. A mis-targeted ad is less consequential than a brand-threatening security breach (one John Smith seeing another John Smith’s personal data); while the former scenario may be lower ROI at the individual level, at high volumes and where some margin of error is acceptable, it would be a strong candidate for probabilistic resolution. Deterministic resolution will continue to be used for identifiers of known and popular systems. But for identifiers we’re not familiar with or can’t resolve with certainty, we will be able to find use cases for probabilistic resolution. Identity resolution is the primary value proposition of a CDP, so the best CDPs will do this very well. Better identity resolution will greatly enhance customer support and other operational use cases, but also spur on personalisation, recommendations and ad campaign optimisation.
6. The Metrics Layer will be the norm and CDPs will benefit from it
This is somewhat a digression but the Metrics Layer is becoming a popular topic these days. It’s a tier that’s envisioned to be wedged between the warehouse and BI tools to provide the “single source of truth” for the definition of metrics. For example, the “number of users” metric may have different definitions depending on the dashboard you look at; the Metrics Layer will provide a single definition of “number of users” for all of these dashboards. You can read about the Metrics Layer in greater, more technical detail in Benn Stancil’s blog.
How does this affect CDPs? If you’ve ever gotten different numbers from Google Analytics and Mixpanel, or Stripe and your eCommerce store, you will know that getting consistent metrics is hard. With CDPs correctly identifying user profiles and the Metrics Layer accurately defining customer metrics, we’ll be able to trust customer insights in our dashboards. And that’s a plus for CDPs, as ill-defined metrics will diminish trust in our analytics and CDP.
7. SQL will be on steroids
SQL has stood the test of time and will continue to be the querying language of choice for extracting customer data. But there’s a limit to SQL. If you’ve ever tried to model a customer journey funnel, you’ll know that it’s a total mess to model funnels with SQL. But we’ll see more tooling that will let us express complex queries more easily. Perhaps a language built on top of SQL that incorporates all the popular language features of SQL but has extra, new expressive features. A Typescript-for-Javascript superset of SQL of sorts. Companies such as Looker have started to make attempts SQL-like iterations of SQL [Malloy by Looker].
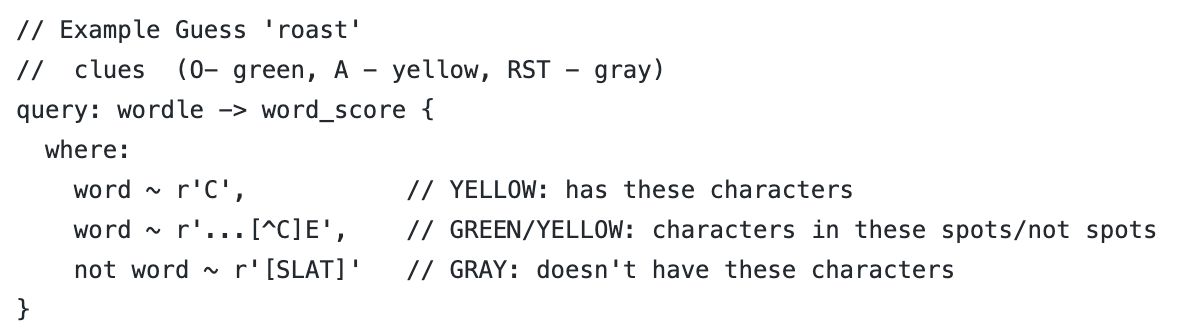
How will this impact CDPs? Easier modelling of customer journeys will mean we can more easily track unique customer journeys. Easier expression of complex queries will make it cheaper to query. We’ll be querying on the go, on our mobile devices, and we’re very likely to see abstractions that will simplify queries for complex customer journeys.
8. DataOps tools will ensure 10x trust in data
It’s essential that data pipelines, error telemetry, monitoring instrumentation and workflows are all in good order for us to garner insights from data. For this to happen, we’ll need very good DataOps tools, so that we can focus on gathering customer insights. DevOps for software engineering is a close analogy: just as we benefited from monitoring and alerting tools, and infrastructure-as-code (IaC) in software, DataOps tooling help data folks focus on data.
There will be direct benefits for CDPs, of course. Bad data erodes trust in our CDP, so it’ll be paramount to detect issues in data quality early and resolve them quickly. Finding out what transformations were applied to a data point before arriving in the dashboard (data lineage) and detecting anomalies will be readily possible in the warehouse-centric world. Data quality will be maintained with correctness (validity), completeness, consistency across sources, credibility and currentness (real-time). DataOps tooling itself will ensure reliability, recoverability and observability across the data infrastructure.
Suppose a bad batch of data resulted in dashboards with incorrect customer data. Reverting changes should be as easy as reverting a commit in a version-controlled code repository. The history of changes made to the data point should be easy to look up (we’re already seeing this in data lineage tools). Perhaps this mess will have been mitigated further by quarantining data in a “dead letter queue” before being applied in the production warehouse. Before even getting ingested into the production infrastructure, we may have tested the data in a staging pipeline, created by a DataOps infrastructure management tool. All of these concerns will impact the CDP and fall under DataOps.
9. Personalised, just like you designed it
We still live in an age where we struggle to personalise users’ content. Sure, we’ve seen big advances in this area through companies such as Qubit, which offer personalised navigation, cart abandonment recovery and personalised product labelling in eCommerce. Klaviyo is now a household name for automating personalised communication with the customer. But we have yet to see hyper-personalisation arrive at scale.
Consider an at-home health care product, for example. Today, you might start the journey by filling out a long health survey, test with the product, send it back for evaluation and then get results explaining your health conditions. Every customer will very likely be presented with a different diagnosis, let alone experience a different journey. Today, a lot of personalisation, if present at all, is based on rules-based logic that presents pre-written content when a certain event is triggered. The holy grail of personalisation is to extract insights in real-time and present the the most relevant copy and interface to the customer. In the future, we’ll see part machine-generated copy and multivariate designs appear on customers’ screens as the CDP works out which stage of the customer journey they’re at.
10. Customer insights at your fingertips, with edge warehouses
Imagine warehouses serving data from the geographically closest CDN server. Warehousing at the edge is already becoming a reality [Edge Intelligence]. You can make a query to the warehouse and get results in milliseconds as the results are already cached at the edge.
Personalisation powered by CDPs will only stand to gain from this. For example, a huge eCommerce website could, in theory, get real-time customer insights without making multiple roundtrips to the data warehouse.
Bringing it all together
So how does a customer experience potentially look like from the CDP’s perspective, in 2030? Let’s consider a highly personalised, at-home gut health test product that has a slightly more complex customer journey than the regular eCommerce product. It’s a contrived example but hopefully it’ll help bring some of the above to life.
- Passively looking: Every customer journey contains a struggle or a desire to make progress in your life (Jobs To Be Done). The first time a potential customer — let’s call her Joanna — becomes aware of their problem is when they encounter their first struggle. This might be adverse effects Joanna is experiencing towards certain foods. She researches online combinations of keywords to describe her ailments. Joanna lands on your blog and an influencer’s vlog who you’ve recently established a partnership with. She’s learning about her problem. The personalisation engine doesn’t have enough data on the customer profile, so a primary call-to-action (CTA) appears on the blogpost created with a No-Code blog builder to sign up to the newsletter. There is also a secondary CTA to visit the product page but we guess that she’s not quite ready to make any decisions yet. She signs up to the newsletter to learn more about gut health.
- Actively looking: Joanna’s been to her practitioner and there’s no clear diagnosis of her problem. So she’s getting a bit frustrated, understandably. She revisits your blog. At this point, she’s still an anonymous user but we can make inferences and associate her previous blog and influencer vlog visits with her profile. The warehouse-centric CDP, available to small startups like ours thanks to serverless, may indicate that probabilistically the blog and vlog visitors are the same. The personalisation engine swaps the primary and secondary CTAs, so that the product page CTA is made more prominent. She visits the product page to see if the product addresses some or all of her problems. Joanna also hearts Tweets you’ve made about links between wheat-based foods and certain ailments.
- Deciding: Joanna decides that the product is going to help her pin-point the root causes of her ailments. Insights stored at the edge show that visitors from Joanna’s country are particularly worried about international shipping. A small banner appears to reassure her the ease of delivery and returns. She buys the product.
- Consuming: The product is dispatched; Joanna finally receives the product, takes the gut health test and registers her product in the online portal. All of these interactions flow through to the warehouse. She sends the test sample back to the company for evaluation. A few days go by. The warehouse — built for marketing and data teams — contains aggregate data on previous email communications and suggests that sending a reassuring email now will put her anxieties at ease. We trust this data as we can trace the lineage of our data points and have confidence in our DataOps. In the meantime, her product registration is registered as an “activation” — as per the Metrics Layer definition — in the dashboard. She receives her test results in a report and learns about her diagnosis. Joanna’s learned something new and expresses her satisfaction with the product in an in-the-moment survey. When she reaches out to support to clarify parts of her report, the support agent has a full picture of her journey as insights from the warehouse are immediately to hand in the help desk tool. Some of these insights — funnel metrics, for example — may have been distilled from expressive “SQL on steroids”.
…a contrived example that brings together all of the elements I discussed above! Of course, mapping out a complex journey is never easy but I have high hopes that by 2030 (or sooner), even the smallest startups will be able to afford and take advantage of the advances being made in the Modern Data Stack. 2030 might seem some ways off but it was only in 2012 when the likes of Segment and Snowflake emerged. What’s to say that we won’t make even bigger leaps by 2030?
Comments