Data is what drives all industries today. From quantitative traders analyzing and forecasting stock market trends to healthcare professionals projecting the severity and virality of newborn pandemics, data is the key factor that is common to everything. Small amounts of data are easy to analyze with a calculator or an excel spreadsheet but once the calculations become complex and the amount of data exponentially grows, we need stronger tools under our belt. This is where software analytics and data science comes in.
Although there are many available software analytic tools today, such as Matlab and R, the one I am focusing in today is Python. Python is very powerful when it comes to data analytics due to the multitude of libraries that many people have built over the years. Although it is important to thoroughly learn as many of these libraries as possible if you would want to pursue a career in data science, I will be going over some beginner libraries that people usually start off with.
NumPy
This is the most fundamental library that all data scientists need to learn. It provides all of the basic functions in scientific computing and is able to process lots of data quickly. The following code is a quick example of what NumPy can do.
The code above will produce the following output:
Input: [0, 1.5707963267948966, 3.141592653589793, 4.71238898038469, 6.283185307179586]Sine values: [ 0.0000000e+00 1.0000000e+00 1.2246468e-16 -1.0000000e+00-2.4492936e-16]Cosine values: [ 1.0000000e+00 6.1232340e-17 -1.0000000e+00 -1.8369702e-161.0000000e+00]Sine values: [ 0. 1. 0. -1. -0.]Cosine values: [ 1. 0. -1. -0. 1.]
Which makes sense from looking at the graph below:

NumPy can also do more complex calculations like the following where it computes complex matrices for a Kalman Filter in self-driving cars:
There is a huge collection of built in functions for all kinds of scientific calculations and if that is what you’re interested in, NumPy is the library to master. To learn more go to the official documentation site for NumPy.
pandas
This is the most fundamental library for data analysis and manipulation in Python. This library is able to quickly read large raw data files into a DataFrame object, perform all kinds of data cleaning and data mining operations with automatic indexing and data alignment, execute all possible SQL queries on the DataFrame table, such as joins and merges, and then output the data into another data file or even directly into visualizations.
What’s really great about using pandas in a Python environment is that Python is a scripting language which means that code can be executed without compiling first. Therefore, data scientists can perform quick analytics through opening up a Python terminal like the following:

With pandas, a data scientist can easily search for data related to a certain client by querying for their clientId and join it with other raw data sets as engineers usually do with databases but now with raw data files. Additionally, data scientists can generate all kinds of visualizations easily with a single line like the following:
df = pd.DataFrame('some data...')df.plot(x='label1', y='label2', kind='scatter', ...)
All in all, pandas provides an easy way to read/write data and makes it very easy and fast to perform analytics on raw data files and is an important tool to have in any data scientist’s arsenal. To learn more about pandas, visit the official documentation site.
SciPy
The SciPy library is an abstracted layer on top of NumPy and the rest of the SciPy stack. This library includes many numerical routines such as numerical integration, interpolation, optimization, linear algebra, statistics, special functions, FFT, signal and image processing, ODE solvers and other tasks common in science and engineering. Therefore, this library is more specific to mathematical functions that tailor towards calculations done by scientists and engineers from an academic standpoint. To learn more, visit the official documentation site.
Scikit-Learn
The Scikit-Learn library is further abstracted on top of SciPy and is more practical and application focused. This library includes functions that focuses more on machine learning applications such as regression, classification, clustering, etc. People who are passionate about machine learning will definitely want to learn more about functionalities that this library provides. For example, you can easily run a RANSAC linear regression on a set of raw data by running the following code:
# generate random data with a small set of outliers
np.random.seed(0)
X[:n_outliers] = 3 + 0.5 * np.random.normal(size=(n_outliers, 1))
y[:n_outliers] = -3 + 10 * np.random.normal(size=n_outliers)
# Fit line using all data
lr = linear_model.LinearRegression()
lr.fit(X, y)
# Robustly fit linear model with RANSAC algorithm
ransac = linear_model.RANSACRegressor()
ransac.fit(X, y)
inlier_mask = ransac.inlier_mask_
outlier_mask = np.logical_not(inlier_mask)
# Predict data of estimated models
line_X = np.arange(X.min(), X.max())[:, np.newaxis]
line_y = lr.predict(line_X)
line_y_ransac = ransac.predict(line_X)

Scikit-Learn also includes a very useful package called preprocessing that is great for data mining and cleaning/sorting out raw data. This package contains functions like normalizations, scaling data to a range, scaling sparse data, and even performing non-linear transformations like mapping data onto a uniform distribution. Additionally, it also has a function that randomly splits data to be used in models:
sklearn.model_selection.train_test_split(*arrays, **options)[source]
Using Scikit-Learn, data scientists can create machine learning models with only a couple of lines. To learn more about Scikit-Learn, visit the official documentation site.
matplotlib.pyplot
This library, although has nothing to do with the analytics portion of data science, is also a key library to learn and use. This library is the Python adaptation of Matlab’s plotting functionality. This library is able to generate anything from simple scatterplots, histograms, line plots to complex heatmaps, 3D plots, eclipses, streamplots, etc. Some examples of these plots are below:

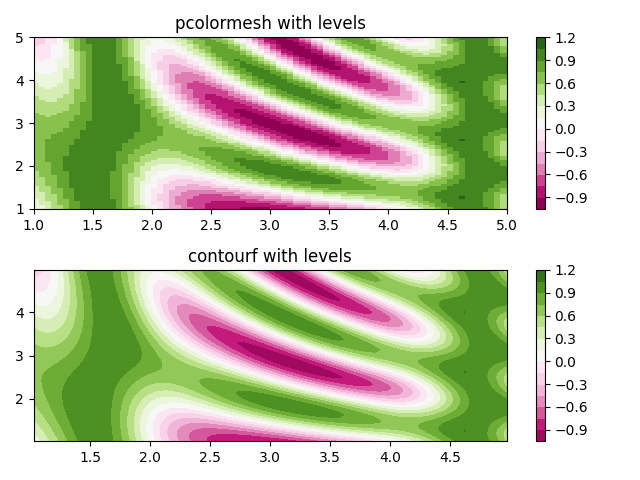
Data Visualization is a huge part of data science because all of the analytics are useless if there isn’t a way to convey the results in an understandable manner. There are definitely better options for data visualization available but most of them are paid or require a subscription. Matplotlib provides a Python native way to visualize data and is definitely something an aspiring analyst should learn.
Conclusion
There are lots of Python libraries that I did not cover. There are lots of libraries targeted towards bio-informatics, deep-learning and AI, self-driving, etc. However, the libraries outlined here are widely used in data science and are the building blocks of many advanced Python libraries. I believe that becoming familiar with these libraries will build up a strong foundation for a beginner who wants to explore the field of data science. If there are any other common Python libraries that you think would be useful to learn, please share them in the comments below.
No comments:
Post a Comment