In the rapidly evolving fields of artificial intelligence (AI), machine learning (ML), and data engineering, the need for efficient data storage and retrieval systems is paramount. Vector databases have emerged as a critical solution for managing the complex, high-dimensional data that these technologies often rely on. Here, we explore seven vector databases that every AI/ML/data engineer should be familiar with, highlighting their unique features and how they support the demands of modern data-driven applications.
1. Milvus
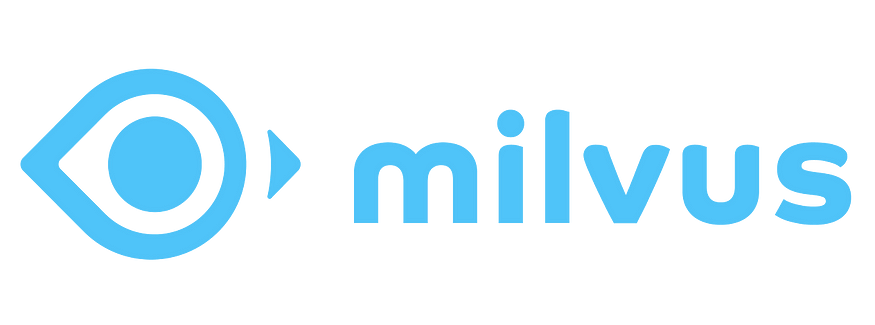
Milvus is an open-source vector database designed to handle large-scale similarity search and vector indexing. It supports multiple index types and offers highly efficient search capabilities, making it suitable for a wide range of AI and ML applications, including image and video recognition, natural language processing, and recommendation systems.
Key Features:
- Highly scalable, supporting billions of vectors.
- Supports multiple metric types for similarity search.
- Easy integration with popular machine learning frameworks.
- Robust and flexible indexing mechanisms.
2. Pinecone

Pinecone is a managed vector database service that simplifies the process of building and scaling vector search applications. It offers a simple API for embedding vector search into applications, providing accurate, scalable similarity search with minimal setup and maintenance.
Key Features:
- Managed service with easy setup and scalability.
- Accurate similarity search with sub-second latencies.
- Supports updates and deletions in real-time.
- Integrates easily with existing data pipelines and ML models.
3. SingleStore Database

SingleStore Database started supporting vector storage as a feature back in 2017 when vector databases were not even a thing.
The robust vector database capabilities of SingleStoreDB are tailored to seamlessly serve AI-driven applications, chatbots, image recognition systems and more. With SingleStoreDB, the necessity for maintaining a dedicated vector database for your vector-intensive workloads becomes obsolete.
Diverging from conventional vector database approaches, SingleStoreDB takes a novel approach by housing vector data within relational tables alongside diverse data types. This innovative amalgamation empowers you to effortlessly access comprehensive metadata and additional attributes pertaining to your vector data, all while leveraging the extensive querying prowess of SQL.
SingleStore’s latest new features for vector search
We are thrilled to announce the arrival of SingleStore Pro Max One of the highlights of the release includes vector search enhancements.
Two important new features have been added to improve vector data processing, and the performance of vector search.
Indexed ANN vector search facilitates creation of large-scale semantic search and generative AI applications. Supported index types include inverted file (IVF), hierarchical navigable small world (HNSW) and variants of both based on product quantization (PQ) — a vector compression method. The VECTOR type makes it easier to create, test, and debug vector-based applications. New infix operators are available for DOT_PRODUCT (<*>) and EUCLIDEAN_DISTANCE (<->) to help shorten queries and make them more readable.
Key Features:
- Real-time analytics and HTAP capabilities for GenAI applications.
- Highly scalable vector store support.
- Scalable, distributed architecture.
- Support for SQL and JSON queries.
- Inbuilt Notebooks feature to work with vector data and GenAI applications.
- Extensible framework for vector similarity search.
4. Weaviate

Weaviate is an open-source vector search engine with out-of-the-box support for vectorization, classification, and semantic search. It is designed to make vector search accessible and scalable, supporting use cases such as semantic text search, automatic classification, and more.
Key Features:
- Automatic machine learning models for data vectorization.
- Semantic search with built-in graph database capabilities.
- Real-time indexing and search.
- GraphQL and RESTful API support.
5. Qdrant

Qdrant is an open-source vector search engine optimized for performance and flexibility. It supports both exact and approximate nearest neighbor search, providing a balance between accuracy and speed for various AI and ML applications.
Key Features:
- Configurable balance between search accuracy and performance.
- Supports payload filtering for advanced search capabilities.
- Real-time data updates and scalable storage.
- Comprehensive API for easy integration.
6. Chroma DB

Chroma DB is a newer entrant in the vector database arena, designed specifically for handling high-dimensional color vectors. It’s particularly useful for applications in digital media, e-commerce, and content discovery, where color similarity plays a crucial role in search and recommendation algorithms.
Key Features:
- Specialized in high-dimensional color vector search.
- Ideal for digital media and e-commerce applications.
- Efficient indexing and retrieval of color data.
- Supports complex color-based query operations.
7. Zilliz

Zilliz is a powerful vector database designed to empower developers and data scientists in building the next generation of AI and search applications. It offers a robust platform for scalable, efficient, and accurate vector search and analytics, supporting a wide array of AI-driven applications.
Key Features:
- Advanced vector search capabilities with high accuracy.
- Scalable architecture for handling large-scale datasets.
- Seamless integration with AI and ML development workflows.
- Supports a variety of vector data types and search algorithms.
Choosing a Vector Database
Choosing the right vector database for your project involves a nuanced understanding of both your application’s specific needs and the unique capabilities of various vector databases. Vector databases are specialized storage systems designed to efficiently handle high-dimensional vector data, which is commonly used in AI and ML applications for tasks such as similarity search, recommendation systems, and natural language processing.
The decision process should consider several critical factors, including the nature of your data, the scale of your operations, the complexity of your queries, integration ease with existing systems, and, importantly, your performance and latency requirements.
Application Type
- Real-time Analytics: SingleStore
- Large-scale Similarity Search: Milvus, Pinecone
- Managed Service: Pinecone
- Hybrid Search: SingleStore
- Semantic Search: Weaviate
- High-dimensional Color Vectors: Chroma DB
Feature Requirements
- Scalability: Milvus, Pinecone, Vald
- Ease of Integration: Weaviate, Zilliz
- Real-time Updates: SingleStore, Qdrant
- Advanced Search Capabilities: Qdrant, Zilliz
Deployment Environment
- On-premises: SingleStore, Milvus
- Cloud: Pinecone, Zilliz
- Hybrid: SingleStore
Performance and Latency
- High Performance: Zilliz
- Low Latency: SingleStore, Pinecone
But, Do you Really Need a Specialised Vector Database?
The hype is all about Generative AI and of course, that has made the vector databases very popular. It is very usual case where we see organizations already juggling between databases for their various use cases. Instead of opting for a specialised vector database, it is always recommended to go for an end-to-end centralised database that can help you with almost all of your use cases — The one that supports real-time analytics, fast, supports all data types, vector storage, etc.
Also, there is a common issue faced by many organizations: The challenge of integrating specialty vector databases into their data architectures, which often results in a variety of operational problems. These problems can include redundant data, excessive data movement, increased labor and licensing costs, and limited query capabilities. Specialty vector databases, while designed to handle specific types of data and workloads (such as vector similarity searches crucial for AI applications), can complicate an organization’s data infrastructure due to these limitations.
SingleStore offers an alternative solution to these challenges. It is a modern database platform that integrates vector database functionality within its broader database system. This integration allows SingleStore to support AI-powered applications, including chatbots, image recognition, and more, without the need for a separate specialty vector database.