Understanding KQL Operators
Let's take a look at Kusto Query Language (KQL) operators and how they can help build powerful queries.
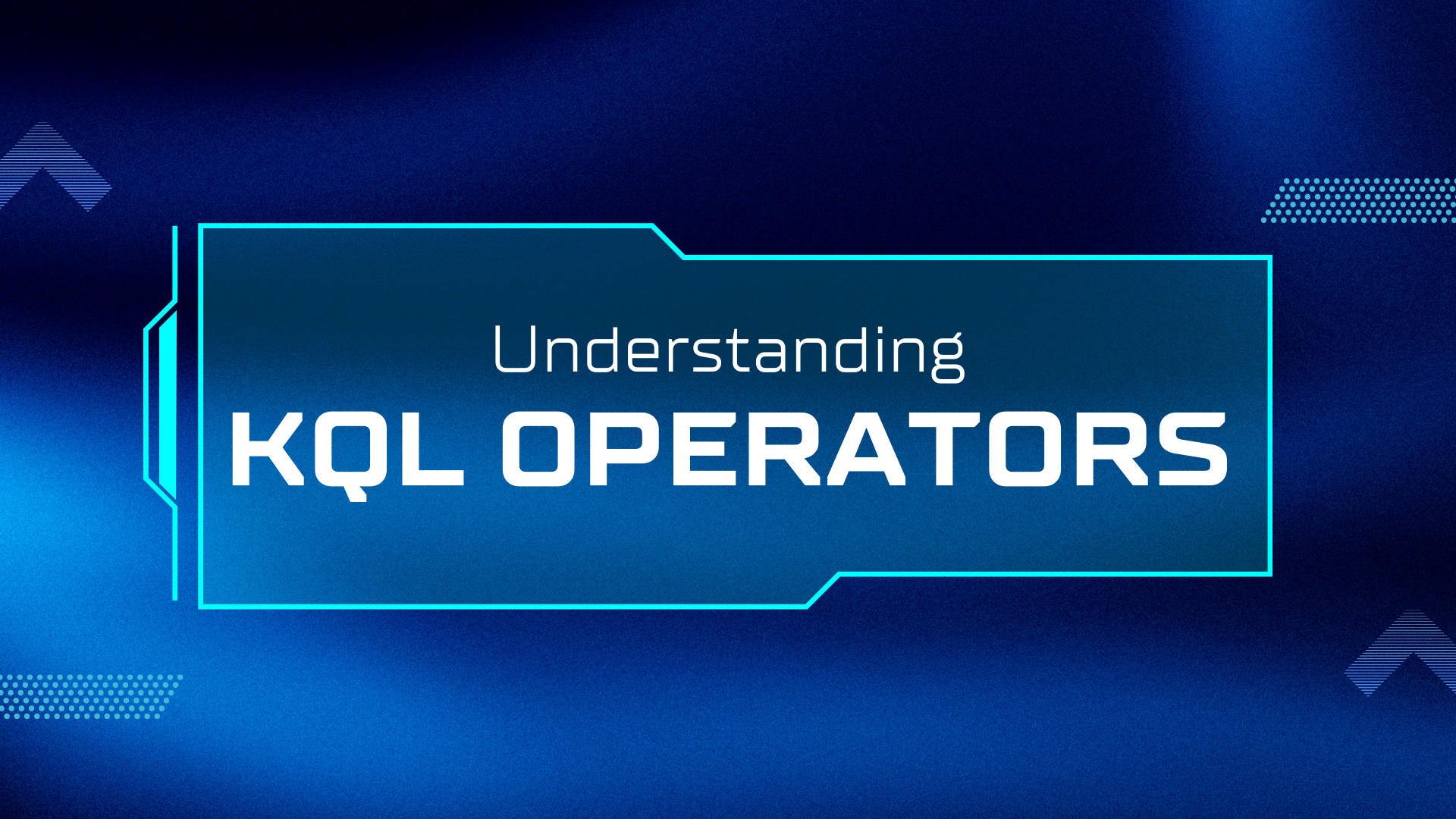
Kusto Query Language (KQL) is a powerful query language that is used in a wide variety of Microsoft products, such as Azure Monitor, Log Analytics, and Microsoft Sentinel.
Like any language, there are some core parts you need to learn to be able to use it effectively.
Below, we’ll explore some of the most commonly used KQL operators and how they can be used to write powerful queries.
KQL Operators
When writing queries in Kusto Query Language (KQL) operators are the building blocks. They allow you to filter, shape, aggregate, and manipulate data efficiently, making it easy to derive insights and identify trends.
Project
The project operator allows you to shape the results of a query output by allowing you to select or rename the columns that appear in the output.
This is useful when you have a dataset that has a lot of columns and you only want to focus on the data from three or four columns.
Summarize
The summarize operator can be used for aggregating data. It performs operations such as counting, averaging, summing or finding minimum and maximum values over specified groups or the entire dataset you are querying.
Using the StormEvents dataset I can summarize how many of each type of storm event happened.
I could also use the summarize operator to calculate the average property damage for each combination of state and event type.
Extend
The extend operator gives you the power to create new columns or modify existing ones. Now remember that KQL is a read-only language, so the extend operator only affects the output results, not the original dataset.
In the example below I am creating a new column by multiplying what is in the “TotalCost” column.
Where
The where filter helps to give you precise results in your query, by only showing rows based on specific conditions.
In the example below, I am filtering so that the State column equals Florida. You can also see I am using the project operator and only showing certain columns.
Distinct
The distinct operator is used to return unique values from a specific column or a combination of columns in a dataset. It effectively removes duplicates, showing only distinct rows based on the columns' specified.
In the example here I only want to see the distinct enters for the EventType in the StormEvents dataset.
Join
The join operator combines rows from two datasets based on a matching condition.
In the example below I am joining the Products and SalesFact tables using the ProductKey column. I am then able to build out the result that shows me the Product name, Product Key, Sales Amount and Total Cost.
Order By
The order by operator can be used to sort the rows of a dataset based on one or more columns in either ascending or descending order. It helps you organise your data for better analysis.
Using the StormEvents dataset again I can order the results of my query based on the DamageProperty column in descending order and project out the columns I need to see.
I can use it multiple times in my query though, I can first group by State in ascending order and then sort by Damage Property in descending order within each state. The result is the output is organised alphabetically by state, and within each state, events are sorted by the highest damage first.
Render
The render operator must be the last operator in a query. It doesn't modify data instead it visualises the data in different forms. There are eleven different visualisations such as area chart, line chart, pie chart or time chart.
Conclusion
These Kusto Query Language (KQL) operators will help you write powerful queries and pull out the data you are looking for within a dataset.
There is a lot to learn with KQL, understanding the operators is just the start. Stay tuned for more KQL insights here!
Sign up for Techielass - A blog by Sarah Lean
Technical…Practical